Developing the Population Health Model
Contents
Developing the Population Health Model#
Overview#
This notebook contains the code to develop the population health model.
Initially 3 different models are compared (Linear regression, Random Forest regresstion, Gradient Boosted regression).
Hyper-parameters of the best model are fine-tuned to maximise performance in unseen data while preventing over-fitting and minimising model complexity
#turn warnings off to keep notebook tidy
import warnings
warnings.filterwarnings('ignore')
Import libraries#
import os
import pandas as pd
import numpy as np
import pickle as pkl
from sklearn.linear_model import LinearRegression
from sklearn.ensemble import RandomForestRegressor
from sklearn.ensemble import GradientBoostingRegressor
from sklearn.model_selection import cross_validate
from sklearn.model_selection import RepeatedKFold
import seaborn as sns
import matplotlib.pyplot as plt
%matplotlib inline
plt.style.use('ggplot')
Import data#
dta = pd.read_csv('https://raw.githubusercontent.com/CharlotteJames/ed-forecast/main/data/master_scaled_new_pop.csv',
index_col=0)
dta.columns = ['_'.join([c.split('/')[0],c.split('/')[-1]])
if '/' in c else c for c in dta.columns]
dta.shape
(1618, 17)
dta.describe()
111_111_offered | 111_111_answered | amb_sys_made | amb_sys_answered | gp_appt_available | ae_attendances_attendances | population | People | Places | Lives | year | %>65 | %<15 | N>65 | N<15 | |
---|---|---|---|---|---|---|---|---|---|---|---|---|---|---|---|
count | 1618.000000 | 1618.000000 | 1618.000000 | 1618.000000 | 1618.000000 | 1618.000000 | 1618.000000 | 1618.000000 | 1618.000000 | 1618.000000 | 1618.000000 | 1618.000000 | 1618.000000 | 1618.000000 | 1618.000000 |
mean | 330.444458 | 286.600228 | 455.922757 | 340.097999 | 4359.178114 | 486.615273 | 63.312790 | 99.208970 | 99.656618 | 100.787827 | 2018.501854 | 18.463638 | 18.056074 | 11.219593 | 11.545206 |
std | 153.095006 | 132.348601 | 353.046416 | 275.127125 | 776.297557 | 275.070544 | 47.620833 | 5.817237 | 2.423154 | 5.738246 | 0.500151 | 3.794561 | 1.548570 | 7.767128 | 9.122457 |
min | 0.707731 | 0.664178 | 141.067451 | 105.089248 | 1299.605358 | 101.447679 | 11.615900 | 84.800000 | 92.500000 | 87.400000 | 2018.000000 | 9.306882 | 14.560279 | 2.154300 | 1.791500 |
25% | 265.012202 | 239.420469 | 207.797181 | 149.382117 | 3878.647249 | 302.149393 | 26.529400 | 94.600000 | 98.000000 | 97.000000 | 2018.000000 | 16.073826 | 16.951092 | 4.781900 | 5.036400 |
50% | 310.420752 | 265.552822 | 269.156617 | 216.466007 | 4376.215538 | 384.680285 | 50.270400 | 100.150000 | 99.550000 | 101.350000 | 2019.000000 | 18.517093 | 18.153428 | 8.342500 | 8.471700 |
75% | 349.608595 | 300.690725 | 628.356628 | 452.675621 | 4829.604169 | 581.037869 | 86.540900 | 103.685714 | 101.400000 | 105.533333 | 2019.000000 | 20.560113 | 19.123772 | 16.364700 | 14.872900 |
max | 1281.625143 | 1206.970308 | 1698.974593 | 1377.465562 | 6937.180617 | 1460.369210 | 210.807400 | 111.200000 | 105.200000 | 113.300000 | 2019.000000 | 27.403900 | 21.763505 | 36.459800 | 42.117100 |
Add random feature#
# Adding random features
rng = np.random.RandomState(0)
rand_var = rng.rand(dta.shape[0])
dta['rand1'] = rand_var
dta.shape
(1618, 18)
Function to aggregate data#
def group_data(data, features):
#ensure no identical points in train and test
grouped = pd.DataFrame()
for pop, group in data.groupby('population'):
#if len(group.lives.unique())>1:
# print('multiple CCG with same population')
ccg_year = pd.Series(dtype='float64')
for f in features:
ccg_year[f] = group[f].unique()[0]
ccg_year['ae_attendances_attendances'] \
= group.ae_attendances_attendances.mean()
grouped = grouped.append(ccg_year, ignore_index=True)
return grouped
Model Comparison#
Features in the dataset that measure population and population health are:
population:
People:
Places:
Lives:
%>65:
Pair plot#
features = ['population',
'People', 'Places',
'Lives', '%>65','rand1']
grouped = group_data(dta, features)
fig = sns.pairplot(grouped.select_dtypes(include=np.number),
kind="reg",
plot_kws={'line_kws':{'color':'black'},
'scatter_kws':
{'color':'green','alpha': 0.1}},
diag_kws={'color':'red'})
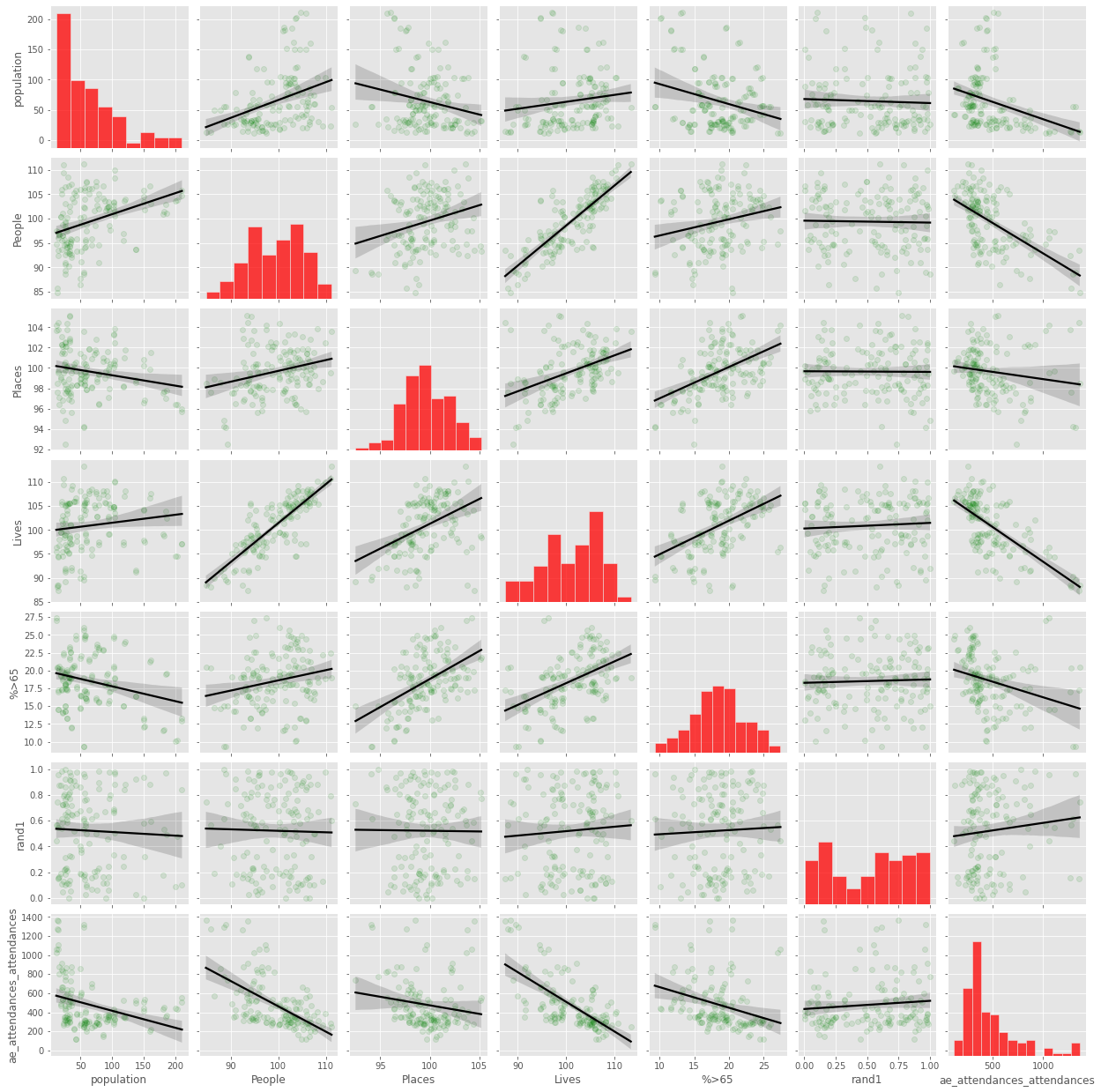
Linear Regression#
model = LinearRegression()
features = ['population',
'People', 'Places',
'Lives', '%>65','rand1']
grouped = group_data(dta, features)
y = grouped['ae_attendances_attendances']
X = grouped[features]
cv = RepeatedKFold(n_splits=5, n_repeats=5, random_state=1)
scores_train, scores_test, feats = [],[],[]
for train_index, test_index in cv.split(X, y):
model.fit(X.iloc[train_index], y.iloc[train_index])
scores_test.append(model.score(X.iloc[test_index],
y.iloc[test_index]))
scores_train.append(model.score(X.iloc[train_index],
y.iloc[train_index]))
feats.append(model.coef_)
Performance#
results=pd.DataFrame()
results['train'] = scores_train
results['test'] = scores_test
results.describe()
train | test | |
---|---|---|
count | 25.000000 | 25.000000 |
mean | 0.552068 | 0.371351 |
std | 0.035222 | 0.343497 |
min | 0.476669 | -1.013811 |
25% | 0.528894 | 0.346533 |
50% | 0.556832 | 0.455708 |
75% | 0.578657 | 0.556435 |
max | 0.613870 | 0.656112 |
Feature Importance#
feat_imp = pd.DataFrame()
for i,f in enumerate(features):
feat_imp[f] = np.array(feats)[:,i]
feat_imp.describe()
population | People | Places | Lives | %>65 | rand1 | |
---|---|---|---|---|---|---|
count | 25.000000 | 25.000000 | 25.000000 | 25.000000 | 25.000000 | 25.000000 |
mean | -1.435290 | 5.844501 | 15.737868 | -34.576050 | -9.079814 | 123.746553 |
std | 0.175190 | 3.047085 | 5.660999 | 4.188117 | 3.984531 | 27.108885 |
min | -1.730007 | 1.434910 | 4.349379 | -43.042262 | -18.441213 | 88.434422 |
25% | -1.513520 | 3.678942 | 9.979621 | -36.339932 | -11.124901 | 102.146007 |
50% | -1.421376 | 5.312968 | 16.724959 | -34.109459 | -9.472548 | 121.696265 |
75% | -1.358518 | 7.613663 | 19.380730 | -32.524713 | -5.919612 | 136.721486 |
max | -0.896284 | 12.445317 | 25.457699 | -25.979856 | -1.672032 | 191.783194 |
Random Forest#
model = RandomForestRegressor(max_depth=4, n_estimators=2,
random_state=0)
y = grouped['ae_attendances_attendances']
X = grouped[features]
cv = RepeatedKFold(n_splits=5, n_repeats=5, random_state=1)
scores_train, scores_test, feats = [],[],[]
for train_index, test_index in cv.split(X, y):
model.fit(X.iloc[train_index], y.iloc[train_index])
scores_test.append(model.score(X.iloc[test_index],
y.iloc[test_index]))
scores_train.append(model.score(X.iloc[train_index],
y.iloc[train_index]))
feats.append(model.feature_importances_)
Performance#
results=pd.DataFrame()
results['train'] = scores_train
results['test'] = scores_test
results.describe()
train | test | |
---|---|---|
count | 25.000000 | 25.000000 |
mean | 0.807108 | 0.565794 |
std | 0.042312 | 0.287908 |
min | 0.720547 | -0.598879 |
25% | 0.776415 | 0.560282 |
50% | 0.809265 | 0.629445 |
75% | 0.836854 | 0.685060 |
max | 0.890000 | 0.834171 |
Feature Importance#
feat_imp = pd.DataFrame()
for i,f in enumerate(features):
feat_imp[f] = np.array(feats)[:,i]
feat_imp.describe()
population | People | Places | Lives | %>65 | rand1 | |
---|---|---|---|---|---|---|
count | 25.000000 | 25.000000 | 25.000000 | 25.000000 | 25.000000 | 25.000000 |
mean | 0.435902 | 0.066185 | 0.062171 | 0.348578 | 0.066302 | 0.020863 |
std | 0.171014 | 0.094662 | 0.052771 | 0.187946 | 0.034714 | 0.021494 |
min | 0.117128 | 0.000000 | 0.000000 | 0.040872 | 0.009537 | 0.000000 |
25% | 0.343149 | 0.011843 | 0.020668 | 0.222201 | 0.041677 | 0.000415 |
50% | 0.419343 | 0.021413 | 0.052408 | 0.349634 | 0.071220 | 0.012603 |
75% | 0.602196 | 0.100430 | 0.093383 | 0.447492 | 0.084021 | 0.030384 |
max | 0.759784 | 0.306692 | 0.223416 | 0.766654 | 0.155546 | 0.075973 |
Gradient boosted tress#
model = GradientBoostingRegressor(max_depth=5, n_estimators=5,
random_state=1)
y = grouped['ae_attendances_attendances']
X = grouped[features]
cv = RepeatedKFold(n_splits=5, n_repeats=5, random_state=1)
scores_train, scores_test, feats = [],[],[]
for train_index, test_index in cv.split(X, y):
model.fit(X.iloc[train_index], y.iloc[train_index])
scores_test.append(model.score(X.iloc[test_index],
y.iloc[test_index]))
scores_train.append(model.score(X.iloc[train_index],
y.iloc[train_index]))
feats.append(model.feature_importances_)
Performance#
results=pd.DataFrame()
results['train'] = scores_train
results['test'] = scores_test
results.describe()
train | test | |
---|---|---|
count | 25.000000 | 25.000000 |
mean | 0.593587 | 0.393527 |
std | 0.014388 | 0.148094 |
min | 0.564452 | -0.128025 |
25% | 0.584104 | 0.316253 |
50% | 0.592172 | 0.401325 |
75% | 0.605975 | 0.493652 |
max | 0.625392 | 0.566740 |
Feature Importance#
feat_imp = pd.DataFrame()
for i,f in enumerate(features):
feat_imp[f] = np.array(feats)[:,i]
feat_imp.describe()
population | People | Places | Lives | %>65 | rand1 | |
---|---|---|---|---|---|---|
count | 25.000000 | 25.000000 | 25.000000 | 25.000000 | 25.000000 | 25.000000 |
mean | 0.432119 | 0.051428 | 0.073296 | 0.326313 | 0.097161 | 0.019683 |
std | 0.196302 | 0.051212 | 0.073320 | 0.229912 | 0.060103 | 0.014576 |
min | 0.099755 | 0.004335 | 0.002015 | 0.033923 | 0.014763 | 0.000250 |
25% | 0.264346 | 0.024739 | 0.017823 | 0.126553 | 0.052532 | 0.005641 |
50% | 0.512337 | 0.036215 | 0.048561 | 0.203326 | 0.091596 | 0.015922 |
75% | 0.604362 | 0.052902 | 0.091070 | 0.519404 | 0.138055 | 0.031943 |
max | 0.718238 | 0.200017 | 0.264029 | 0.693263 | 0.266472 | 0.049490 |
Summary#
Logistic Regression
Variable performance with different splits: mean \(R^2\) = 0.4, minimum \(R^2\) = -0.5 in test set
Random Forest
Best performance with mean \(R^2\) = 0.5 in test data.
Performance also variable: minimum \(R^2\) = -0.8
Feature importance is stable: population is most important, followed by Lives, People then Places.
The random feature has low importnace which validates the importance of other features.
Gradient Boosted Trees
Doesn’t perform as well as a Random Forest, mean \(R^2\) = 0.4 in test data
Performance also variable: minimum \(R^2\) = -0.24
Feature importance is not agreement with the Random Forest, with Places more important than People.
Hyper parameter tuning#
The best model is the Random Forest. To ensure the model is not over fit to the training data we compare performance when the following parameters are varied:
max_depth: the maximum size of any tree
n_estimators: the number of trees in the forest
Maximum Depth#
d = [1,2,3,4,5,6,7,8,9]
res_train,res_test = [],[]
for depth in d:
model = RandomForestRegressor(max_depth=depth,
n_estimators=3, random_state=0)
y = grouped['ae_attendances_attendances']
X = grouped[features]
cv = RepeatedKFold(n_splits=5, n_repeats=5, random_state=1)
scores_train, scores_test = [],[]
for train_index, test_index in cv.split(X, y):
model.fit(X.iloc[train_index], y.iloc[train_index])
scores_test.append(model.score(X.iloc[test_index],
y.iloc[test_index]))
scores_train.append(model.score(X.iloc[train_index]
, y.iloc[train_index]))
res_train.append(scores_train)
res_test.append(scores_test)
Plot#
fig,ax = plt.subplots(figsize=(8,5))
plt.plot(d, np.mean(res_train, axis=1), 'b--', label='train')
plt.plot(d, np.mean(res_test, axis=1), 'r--', label='test')
plt.fill_between(d, y1=(np.mean(res_train, axis=1)-np.std(res_train, axis=1)),
y2=(np.mean(res_train, axis=1)+np.std(res_train, axis=1)),
color='b', alpha=0.2)
plt.fill_between(d, y1=(np.mean(res_test, axis=1)-np.std(res_test, axis=1)),
y2=(np.mean(res_test, axis=1)+np.std(res_test, axis=1)),
color='r', alpha=0.2)
plt.legend(loc='best')
plt.xlabel('Maximum Tree Depth')
plt.ylabel('Model performance')
plt.show()
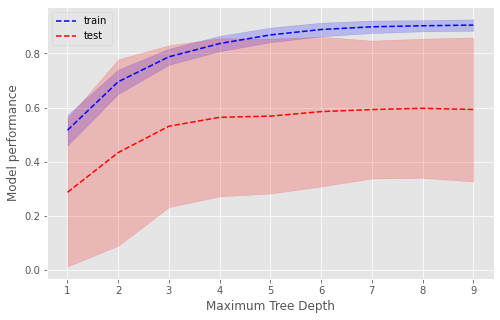
A depth of 7 is optimal. After this, there is no improvement in performance on unseen data (test, red dashed line) and performance continues to increase in the training data (blue dashed line) suggesting overfitting.
Number of Trees#
n = [1,2,3,4,5,6,7]
res_train,res_test = [],[]
for est in n:
model = RandomForestRegressor(max_depth=7, n_estimators=est,
random_state=0)
y = grouped['ae_attendances_attendances']
X = grouped[features]
cv = RepeatedKFold(n_splits=5, n_repeats=5, random_state=1)
scores_train, scores_test = [],[]
for train_index, test_index in cv.split(X, y):
model.fit(X.iloc[train_index], y.iloc[train_index])
scores_test.append(model.score(X.iloc[test_index],
y.iloc[test_index]))
scores_train.append(model.score(X.iloc[train_index],
y.iloc[train_index]))
res_train.append(scores_train)
res_test.append(scores_test)
Plot#
fig,ax = plt.subplots(figsize=(8,5))
plt.plot(n, np.mean(res_train, axis=1), 'b--', label='train')
plt.plot(n, np.mean(res_test, axis=1), 'r--', label='test')
plt.fill_between(n, y1=(np.mean(res_train, axis=1)-np.std(res_train, axis=1)),
y2=(np.mean(res_train, axis=1)+np.std(res_train, axis=1)),
color='b', alpha=0.2)
plt.fill_between(n, y1=(np.mean(res_test, axis=1)-np.std(res_test, axis=1)),
y2=(np.mean(res_test, axis=1)+np.std(res_test, axis=1)),
color='r', alpha=0.2)
plt.legend(loc='best')
plt.xlabel('Number of Trees')
plt.ylabel('Model performance')
plt.show()
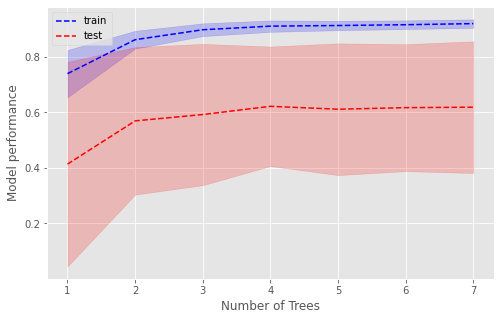
The optimal number of trees is 4, beyond which there is no improvement in the training or test set.
Final Model for paper#
Fit the Random forest with optimal parameters
model = RandomForestRegressor(max_depth=7, n_estimators=4,
random_state=0)
y = grouped['ae_attendances_attendances']
X = grouped[features]
cv = RepeatedKFold(n_splits=5, n_repeats=5, random_state=1)
scores_train, scores_test, feat = [],[],[]
for train_index, test_index in cv.split(X, y):
model.fit(X.iloc[train_index], y.iloc[train_index])
scores_test.append(model.score(X.iloc[test_index],
y.iloc[test_index]))
scores_train.append(model.score(X.iloc[train_index],
y.iloc[train_index]))
feat.append(model.feature_importances_)
Performance#
results=pd.DataFrame()
results['train'] = scores_train
results['test'] = scores_test
results.describe()
train | test | |
---|---|---|
count | 25.000000 | 25.000000 |
mean | 0.911347 | 0.622299 |
std | 0.021012 | 0.219899 |
min | 0.866569 | 0.066347 |
25% | 0.902049 | 0.552788 |
50% | 0.914108 | 0.681962 |
75% | 0.926453 | 0.793663 |
max | 0.938922 | 0.874207 |
Feature Importance#
feat_imp = pd.DataFrame()
for i,f in enumerate(features):
feat_imp[f] = np.array(feats)[:,i]
feat_imp.describe()
population | People | Places | Lives | %>65 | rand1 | |
---|---|---|---|---|---|---|
count | 25.000000 | 25.000000 | 25.000000 | 25.000000 | 25.000000 | 25.000000 |
mean | 0.432119 | 0.051428 | 0.073296 | 0.326313 | 0.097161 | 0.019683 |
std | 0.196302 | 0.051212 | 0.073320 | 0.229912 | 0.060103 | 0.014576 |
min | 0.099755 | 0.004335 | 0.002015 | 0.033923 | 0.014763 | 0.000250 |
25% | 0.264346 | 0.024739 | 0.017823 | 0.126553 | 0.052532 | 0.005641 |
50% | 0.512337 | 0.036215 | 0.048561 | 0.203326 | 0.091596 | 0.015922 |
75% | 0.604362 | 0.052902 | 0.091070 | 0.519404 | 0.138055 | 0.031943 |
max | 0.718238 | 0.200017 | 0.264029 | 0.693263 | 0.266472 | 0.049490 |