Separating Capacity and Utility
Contents
Separating Capacity and Utility#
Overview#
The capacity and utility features are highly correlated (see below). This can affect overall model performance and feature importances.
To understand the extent of this effect we fit separate models to capacity and utility features, and compare performance.
Capacity features: 111 calls offered, Ambulance answered, GP appointments available
Utility features: 111 calls answered, Ambulance made
#turn warnings off to keep notebook tidy
import warnings
warnings.filterwarnings('ignore')
Import libraries#
import os
import pandas as pd
import numpy as np
from sklearn.ensemble import RandomForestRegressor
from sklearn.ensemble import GradientBoostingRegressor
from sklearn.linear_model import LinearRegression
from sklearn.model_selection import train_test_split
from sklearn.pipeline import make_pipeline
from sklearn.preprocessing import StandardScaler
from sklearn.model_selection import cross_validate
from sklearn.model_selection import RepeatedKFold
import matplotlib.pyplot as plt
%matplotlib inline
plt.style.use('ggplot')
Import data#
dta = pd.read_csv('https://raw.githubusercontent.com/CharlotteJames/ed-forecast/main/data/master_scaled_new.csv',
index_col=0)
dta.columns = ['_'.join([c.split('/')[0],c.split('/')[-1]])
if '/' in c else c for c in dta.columns]
dta.ccg.unique().shape
(74,)
Feature correlations#
fig,ax_list = plt.subplots(1,2, figsize = (10,5))
xx = np.arange(0,140)*10
ax = ax_list[0]
ax.plot(dta['111_111_answered'].values, dta['111_111_offered'].values, 'ro')
ax.set_xlabel('111 Utility')
ax.set_ylabel('111 Capacity')
ax.plot(xx,xx, 'k--')
ax = ax_list[1]
ax.plot(dta['amb_sys_made'].values, dta['amb_sys_answered'].values, 'bo')
ax.set_xlabel('Ambulance Utility')
ax.set_ylabel('Ambulance Capacity')
ax.plot(xx,xx,'k--')
plt.show()
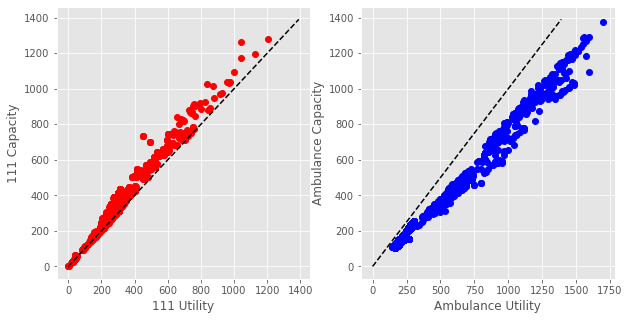
Add random feature#
# Adding random features
rng = np.random.RandomState(0)
rand_var = rng.rand(dta.shape[0])
dta['rand1'] = rand_var
dta.shape
(1618, 14)
Fitting function#
def fit_model(dta, model, features):
y = dta['ae_attendances_attendances']
X = dta[features]
#cross validate to get errors on performance and coefficients
cv_model = cross_validate(model, X,y,
cv=RepeatedKFold(n_splits=5, n_repeats=5,
random_state=0),
return_estimator=True,
return_train_score=True, n_jobs=2)
clf = model.fit(X, y)
return cv_model
Utility Model#
features = ['111_111_answered', 'amb_sys_made', 'rand1']
Linear Regression#
model = LinearRegression()
results = fit_model(dta,model,features)
Performance#
res=pd.DataFrame()
res['test_score'] = results['test_score']
res['train_score'] = results['train_score']
res.describe()
test_score | train_score | |
---|---|---|
count | 25.000000 | 25.000000 |
mean | 0.083855 | 0.089997 |
std | 0.023747 | 0.004965 |
min | 0.046392 | 0.074933 |
25% | 0.072955 | 0.087500 |
50% | 0.084371 | 0.089937 |
75% | 0.096630 | 0.093126 |
max | 0.161442 | 0.100655 |
Coefficients#
coefs = pd.DataFrame(
[model.coef_
for model in results['estimator']],
columns=features
)
coefs.describe()
111_111_answered | amb_sys_made | rand1 | |
---|---|---|---|
count | 25.000000 | 25.000000 | 25.000000 |
mean | 0.388187 | -0.136642 | -3.708228 |
std | 0.016808 | 0.007403 | 12.305010 |
min | 0.339519 | -0.149784 | -25.111645 |
25% | 0.380248 | -0.142231 | -9.562033 |
50% | 0.388083 | -0.135624 | -4.667144 |
75% | 0.395557 | -0.132207 | 4.321049 |
max | 0.427379 | -0.121675 | 19.347927 |
Random Forest#
model = RandomForestRegressor()
results = fit_model(dta,model,features)
Performance#
res=pd.DataFrame()
res['test_score'] = results['test_score']
res['train_score'] = results['train_score']
res.describe()
test_score | train_score | |
---|---|---|
count | 25.000000 | 25.000000 |
mean | 0.236590 | 0.894535 |
std | 0.066466 | 0.003297 |
min | 0.112391 | 0.888562 |
25% | 0.197762 | 0.892035 |
50% | 0.243202 | 0.894518 |
75% | 0.279811 | 0.897373 |
max | 0.331515 | 0.901216 |
Coefficients#
coefs = pd.DataFrame(
[model.feature_importances_
for model in results['estimator']],
columns=features
)
coefs.describe()
111_111_answered | amb_sys_made | rand1 | |
---|---|---|---|
count | 25.000000 | 25.000000 | 25.000000 |
mean | 0.207987 | 0.440134 | 0.351879 |
std | 0.005848 | 0.009332 | 0.009254 |
min | 0.195764 | 0.424368 | 0.326420 |
25% | 0.205005 | 0.433463 | 0.347748 |
50% | 0.207886 | 0.440542 | 0.351117 |
75% | 0.211373 | 0.444091 | 0.357582 |
max | 0.221227 | 0.465251 | 0.368511 |
Gradient Boosted Trees#
model = GradientBoostingRegressor()
results = fit_model(dta,model,features)
Performance#
res=pd.DataFrame()
res['test_score'] = results['test_score']
res['train_score'] = results['train_score']
res.describe()
test_score | train_score | |
---|---|---|
count | 25.000000 | 25.000000 |
mean | 0.337244 | 0.493172 |
std | 0.038370 | 0.009531 |
min | 0.246938 | 0.475122 |
25% | 0.305860 | 0.488163 |
50% | 0.347487 | 0.493584 |
75% | 0.369488 | 0.497437 |
max | 0.387623 | 0.512314 |
Coefficients#
coefs = pd.DataFrame(
[model.feature_importances_
for model in results['estimator']],
columns=features
)
coefs.describe()
111_111_answered | amb_sys_made | rand1 | |
---|---|---|---|
count | 25.000000 | 25.000000 | 25.000000 |
mean | 0.196963 | 0.671507 | 0.131530 |
std | 0.009310 | 0.014289 | 0.010002 |
min | 0.180775 | 0.638809 | 0.114408 |
25% | 0.190780 | 0.660383 | 0.124134 |
50% | 0.197679 | 0.673083 | 0.130621 |
75% | 0.204245 | 0.681347 | 0.137798 |
max | 0.211885 | 0.697861 | 0.149508 |
Summary#
Linear regression performs poorly, with an \(R^2\) < 0.1
Random Forest overfits to the training data, and the random variable is comparatively important
Gradient boosted trees performs best and does not overfit. The random variable is ranked lowest.
Capacity Model#
features = ['gp_appt_available',
'111_111_offered', 'amb_sys_answered', 'rand1']
Linear Regression#
model = LinearRegression()
results = fit_model(dta,model,features)
Performance#
res=pd.DataFrame()
res['test_score'] = results['test_score']
res['train_score'] = results['train_score']
res.describe()
test_score | train_score | |
---|---|---|
count | 25.000000 | 25.000000 |
mean | 0.102073 | 0.108765 |
std | 0.026177 | 0.005678 |
min | 0.052969 | 0.092187 |
25% | 0.088854 | 0.105822 |
50% | 0.104882 | 0.108816 |
75% | 0.113505 | 0.112577 |
max | 0.188438 | 0.122830 |
Coefficients#
coefs = pd.DataFrame(
[model.coef_
for model in results['estimator']],
columns=features
)
coefs.describe()
gp_appt_available | 111_111_offered | amb_sys_answered | rand1 | |
---|---|---|---|---|
count | 25.000000 | 25.000000 | 25.000000 | 25.000000 |
mean | 0.009613 | 0.481967 | -0.108094 | -1.058362 |
std | 0.003273 | 0.012217 | 0.009119 | 12.414753 |
min | 0.002709 | 0.451203 | -0.125017 | -21.694177 |
25% | 0.007641 | 0.478179 | -0.115796 | -7.409371 |
50% | 0.010039 | 0.481001 | -0.107188 | -2.177476 |
75% | 0.011166 | 0.487988 | -0.101752 | 8.037063 |
max | 0.016756 | 0.509044 | -0.089849 | 21.684858 |
Random Forest#
model = RandomForestRegressor()
results = fit_model(dta,model,features)
Performance#
res=pd.DataFrame()
res['test_score'] = results['test_score']
res['train_score'] = results['train_score']
res.describe()
test_score | train_score | |
---|---|---|
count | 25.000000 | 25.000000 |
mean | 0.380144 | 0.914196 |
std | 0.064979 | 0.003352 |
min | 0.232751 | 0.908657 |
25% | 0.335715 | 0.912098 |
50% | 0.389321 | 0.913890 |
75% | 0.425642 | 0.916405 |
max | 0.510369 | 0.921425 |
Coefficients#
coefs = pd.DataFrame(
[model.feature_importances_
for model in results['estimator']],
columns=features
)
coefs.describe()
gp_appt_available | 111_111_offered | amb_sys_answered | rand1 | |
---|---|---|---|---|
count | 25.000000 | 25.000000 | 25.000000 | 25.000000 |
mean | 0.239598 | 0.189017 | 0.401606 | 0.169778 |
std | 0.005962 | 0.011403 | 0.013790 | 0.007099 |
min | 0.229238 | 0.167500 | 0.378487 | 0.150998 |
25% | 0.234416 | 0.181186 | 0.392606 | 0.164699 |
50% | 0.239503 | 0.187306 | 0.400261 | 0.170325 |
75% | 0.243710 | 0.197662 | 0.410551 | 0.173957 |
max | 0.251220 | 0.216889 | 0.437289 | 0.183189 |
Gradient Boosted Trees#
model = GradientBoostingRegressor()
results = fit_model(dta,model,features)
Performance#
res=pd.DataFrame()
res['test_score'] = results['test_score']
res['train_score'] = results['train_score']
res.describe()
test_score | train_score | |
---|---|---|
count | 25.000000 | 25.000000 |
mean | 0.401899 | 0.565627 |
std | 0.050106 | 0.009447 |
min | 0.310316 | 0.549283 |
25% | 0.369650 | 0.556523 |
50% | 0.405141 | 0.567855 |
75% | 0.437094 | 0.573057 |
max | 0.491685 | 0.584443 |
Coefficients#
coefs = pd.DataFrame(
[model.feature_importances_
for model in results['estimator']],
columns=features
)
coefs.describe()
gp_appt_available | 111_111_offered | amb_sys_answered | rand1 | |
---|---|---|---|---|
count | 25.000000 | 25.000000 | 25.000000 | 25.000000 |
mean | 0.161047 | 0.182368 | 0.598112 | 0.058473 |
std | 0.011149 | 0.014722 | 0.016180 | 0.007970 |
min | 0.136198 | 0.153994 | 0.561232 | 0.043424 |
25% | 0.156540 | 0.175966 | 0.589252 | 0.053474 |
50% | 0.161440 | 0.180021 | 0.599186 | 0.057511 |
75% | 0.168531 | 0.189533 | 0.608987 | 0.062670 |
max | 0.184913 | 0.227816 | 0.625746 | 0.075962 |
Summary#
Linear regression again performs poorly.
Random Forest overfits to the training data, but the random variable is ranked lowest.
Gradient boosted trees performs best and does not overfit. The random variable is ranked lowest.